QMNET Talk: Missing Data Imputation with Low Rank Models (Video recording available)
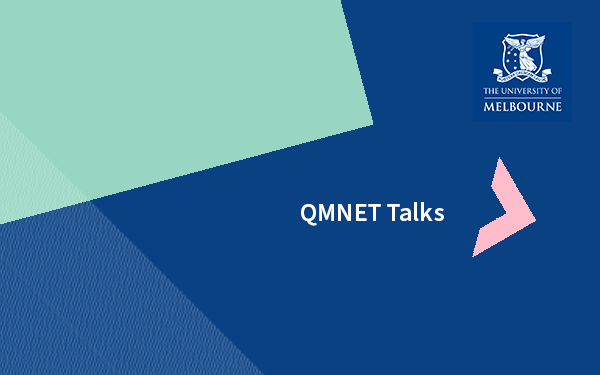
Melbourne Centre for Data Science is proud to support the University of Melbourne Quantitative Research Methods Network (QMNET).
QMNET ran weekly talks on a variety of topics that span disciplinary boundaries.
This talk was by A/Prof Madeleine Udell from Cornell University. She has a BS in Mathematics and Physics from Yale and a PhD in Computational & Mathematical Engineering from Stanford University, and subsequently held a postdoctoral fellowship in the Center for the Mathematics of Information at Caltech. She is now an Assistant Professor of Operations Research and Information Engineering and the Richard and Sybil Smith Sesquicentennial Fellow at Cornell University. Find out more about A/Prof Udell on her site.
Talk Title: Missing Data Imputation with Low Rank Models
Abstract:
Data scientists are often faced with the challenge of understanding a high dimensional data set organised as a table. These tables may have columns of different (sometimes, non-numeric) types, and often have many missing entries. In this talk, we discuss how to use low rank models to analyse these big messy data sets. Low rank models perform well --- indeed, suspiciously well --- across a wide range of data science applications, including applications in social science, medicine, and machine learning. In this talk, we introduce the mathematics of low rank models, demonstrate a few surprising applications of low rank models in data science, and present a simple mathematical explanation for their effectiveness.